Noa Marom, Associate Professor, Department of Materials Science and Engineering, Carnegie Mellon University
Applications of Machine Learning in First-Principles Materials Simulations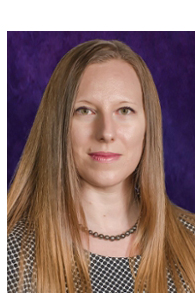
The Department of Chemistry Presents, via Online Zoom Presentation: Dr. Noa Marom, Associate Professor, Department of Materials Science and Engineering, Carnegie Mellon University
In recent years, machine learning (ML) algorithms have been used increasingly in conjunction with first-principles simulations to accelerate computational design and discovery of materials. In this seminar, I will discuss two applications of ML in materials simulations.
The first application is a machine learned model for predicting the volume of a molecular crystal based on the single molecule structure. Predicting the structure and properties of molecular crystal polymorphs is vital across industries whose products are marketed in the form of molecular crystals, primarily the pharmaceutical industry. Computational crystal structure prediction (CSP) has become a standard component of the drug development process. CSP is a formidable challenge because it requires searching a high-dimensional space with high accuracy. The target unit cell volume range defines the region of configuration space to be searched. An accurate estimate of the target volume is essential to the success of CSP. We have developed a volume estimation model based on two descriptors: the volume enclosed by the molecule’s packing-accessible surface and molecular topological fragments. The model is trained on a dataset of structures extracted from the Cambridge Structural Database. Excellent performance is demonstrated for three validation sets of unseen data. The accuracy and robustness of the model may be attributed to including both geometric and chemical features.
The second application is machine learning predictive models for singlet fission. Singlet fission (SF), the conversion of one singlet exciton into two triplet excitons, could significantly enhance solar cell efficiency. Molecular crystals that undergo SF are scarce. Computational exploration may accelerate the discovery of SF materials. However, many-body perturbation theory (MBPT) calculations of the excitonic properties of molecular crystals are impractical for large-scale materials screening. We use the sure-independence-screening-and-sparsifying-operator (SISSO) machine-learning algorithm to generate computationally efficient models that can predict the MBPT thermodynamic driving force for SF for a dataset of 101 polycyclic aromatic hydrocarbons (PAH101). SISSO generates models by iteratively combining physical primary features. The best models are selected by linear regression with cross validation. The SISSO models successfully predict the SF driving force with errors below 0.2 eV. Based on the cost, accuracy, and classification performance of SISSO models, we propose a hierarchical materials screening workflow. Three potential SF candidates are found in the PAH101 set.
[1] I. Bier and N. Marom, J. Phys. Chem. A 124, 10330 (2020)
[2] X. Liu, X. Wang, S. Gao, V. Chang, R. Tom, M. Yu, L. Ghiringhelli, N. Marom, npj Computational
Materials, DOI: 10.1038/s41524-022-00758-y (2022)
Research Area: Computational Materials Science
Noa Marom received a B.A. in Physics and a B.S. in Materials Engineering, both Cum Laude, from the Technion- Israel Institute of Technology in 2003. From 2002 to 2004 she worked as an Application Engineer in the Process Development and Control Division of Applied Materials. In 2010 she received a Ph.D. in Chemistry from the Weizmann Institute of Science. She was awarded the Shimon Reich Memorial Prize of Excellence for her thesis. She then pursued postdoctoral research at the Institute for Computational Engineering and Sciences (ICES) at the University of Texas at Austin. From 2013 to 2016 she was an Assistant Professor in the Physics and Engineering Physics (PEP) Department at Tulane University. In 2016 she joined the Materials Science and Engineering Department at Carnegie Mellon University as an Assistant Professor. She holds courtesy appointments in the Department of Chemistry and the Department of Physics. She is a member of the Pittsburgh Quantum Institute (PQI) and an affiliate of the Scott Institute for Energy Innovation. She has recently received the NSF CAREER, DOE INCITE (2017,2018), and Charles E. Kaufman Young Investigator awards. In 2018 she was awarded the IUPAP Young Scientist Prize in Computational Physics.
nmaromandrew [dot] cmu [dot] edu (nmarom[at]andrew[dot]cmu[dot]edu)